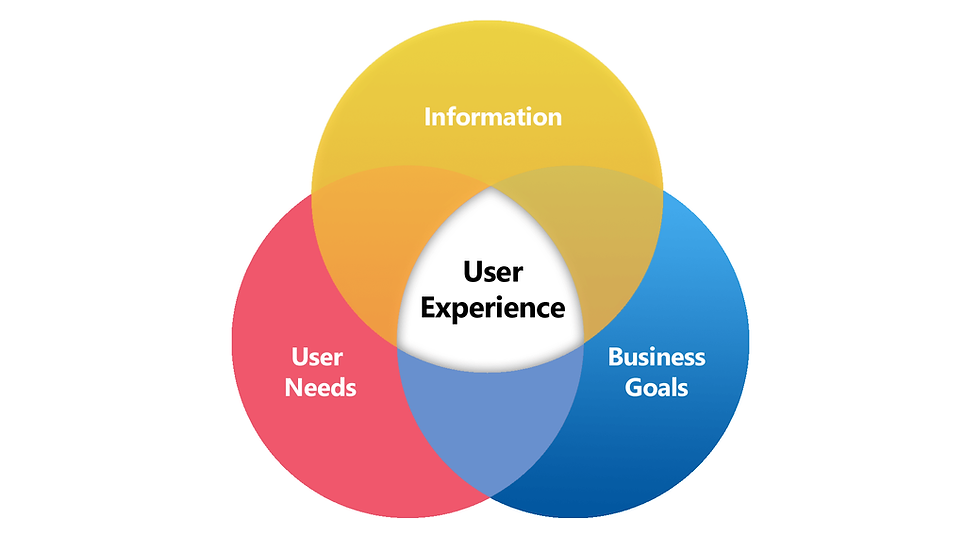
Let’s Talk Numbers
Let’s assume that you have two color options – ‘A’ and ‘B’. You run a survey for 20 users and ask them to rate both the options on a scale of 1 to 10. Now let’s assume ‘A’ got 6 points on average and ‘B’ got 5.2. Would you then choose option ‘A’ because it got a higher rating than ‘B’?
In a usability study, 62% of the users were able to complete a task. Is this a good number?
There’s a 20% increase in the number of visitors to a website. Is that a significant rise in visitors?
When we present stakeholders with findings, they want to see numbers and graphs. If we back our statements with numbers, the audience is more likely to be impressed. However, in many of cases, the data gathered is not scientific and has minimal to zero references. Here’s where we must ask ourselves a few questions: what data should we collect and how should we analyze it to create scientific and statistical significance? Do we just hold our thumb up to the wind to arrive at a magic number or are there some defined methods and tools that we should use to arrive at meaningful statistics? And, how exactly do we do this?
Now that we have identified the right questions, let’s try and find the answers.
Understanding What Numerical Data We Can Collect
Quantitative measures are an indirect assessment of the UX design and are generally either based on the users’ performance of a given task or their perception. We can collect data by using either objective or subjective measures.
Objective data measures
Number of clicks: Measure the number of clicks for the key tasks and check if your design can reduce them. It is believed that the lower the clicks, the better the results but this may not always hold true. In some cases, we may increase the number of clicks by design to improve learnability.
Efficiency: Measuring the time required to complete tasks provides statistical data about the efficiency of the system. Generally, this is associated with the system’s performance. In case of a website (especially on mobile), if it doesn’t load in 3-4 seconds, users will leave. The design also plays an important role in improving performance/efficiency. You need to design screens that will load faster.
Effectiveness: Measuring task completion rate provides statistical data for effectiveness. The higher the rate, the better it is.
Number of assists: Conduct a usability test on your product and measure the number of assists in each task – a lower number of assists is always better for a design.
Number of errors: In usability testing, it’s important to measure the number of errors users have made – the lower, the better.
Subjective data measures
Satisfaction questionnaire: Companies should ideally create their own questionnaires rather than using standard ones. While the latter provides the required score and subjective data, the questionnaire still needs to be statistically reliable.
A few examples: Software Usability Measurement Inventory (SUMI) is one of the standard questionnaires with a subjective score. Another one is the Software Usability Scale (SUS), that provides a score. Generally, 68 is the minimum that your design must score.
What Do We Do with This Data?
Quantitative metrics are pure numbers. However, we cannot make an inference by simply looking at them. Hence, we need to set a benchmark to analyze them better. In UX terms we call this a ‘Usability Matrix’. You need to define what is important for your product. For example, if you want to improve the efficiency of the system, you should conduct an A/B test on your older and new product/process. You must record the task completion time and number of clicks for key tasks. Once done, there needs to a comparison between both the findings to check if the newer product/process has shown significant improvement over the older. This brings us to the next step – determining statistical significance.
What is Statistical Significance?
Statistical significance is a way of mathematically proving the reliability of the study. The statistical significance level reflects the risk tolerance and confidence level. For example, if you run an A/B testing experiment with a significance level of 95%, this means that if you take a decision, you can be 95% confident that the observed results are real and not just an error caused by randomness. It also means that there is a 5% chance that you could be wrong.
The statistical significance is calculated by hypothesis testing. The idea is to define your hypothesis with an appropriate matrix and test it. The most critical part of the process is to have a good hypothesis which you can test. Once you get that, mathematics can help you to get results.
Is Only Quantitative Data Enough?
One of the issues with quantitative data is that it does not indicate what UX problems users have encountered. However, it does tell you where the users have struggled. This will ultimately help designers identify the exact problem and provide the appropriate design solution.
I recommend collecting both qualitative and quantitative data, as this has dual benefits. It helps in discovering real UX issues and in turn provides the right design solution. Additionally, supporting the hypothesis with numbers helps better convince the stakeholders.
A good design is as much science as it is an art. We hope you enjoyed reading a little bit about the intricacies of the process.
Read other Extentia Blog posts here!
EPTU Machine ETPU Moulding…
EPTU Machine ETPU Moulding…
EPTU Machine ETPU Moulding…
EPTU Machine ETPU Moulding…
EPTU Machine ETPU Moulding…
EPS Machine EPS Block…
EPS Machine EPS Block…
EPS Machine EPS Block…
AEON MINING AEON MINING
AEON MINING AEON MINING
KSD Miner KSD Miner
KSD Miner KSD Miner
BCH Miner BCH Miner
BCH Miner BCH Miner
The article helps me better understand how to evaluate user experience scientifically. However, I think more practical examples are needed to illustrate the metrics and methods mentioned. Thanks for sharing! rice purity test meaning