The Fascinating Generative AI Journey: How Does it Work?
- Nisha Sashidharan, Head of Marketing
- Sep 20, 2023
- 6 min read
In the world of Artificial Intelligence (AI), Generative AI is a game-changer. It goes beyond traditional AI, allowing machines to analyze, decide, and create new things. It is important because it sparks artificial creativity, enabling us to generate content like text, images, and music. This blog post is a step-by-step exploration of how Generative AI works, and we will take a quick trip through its history, from its humble beginnings in the 1930s, to modern-day 2023, to better understand its potential.
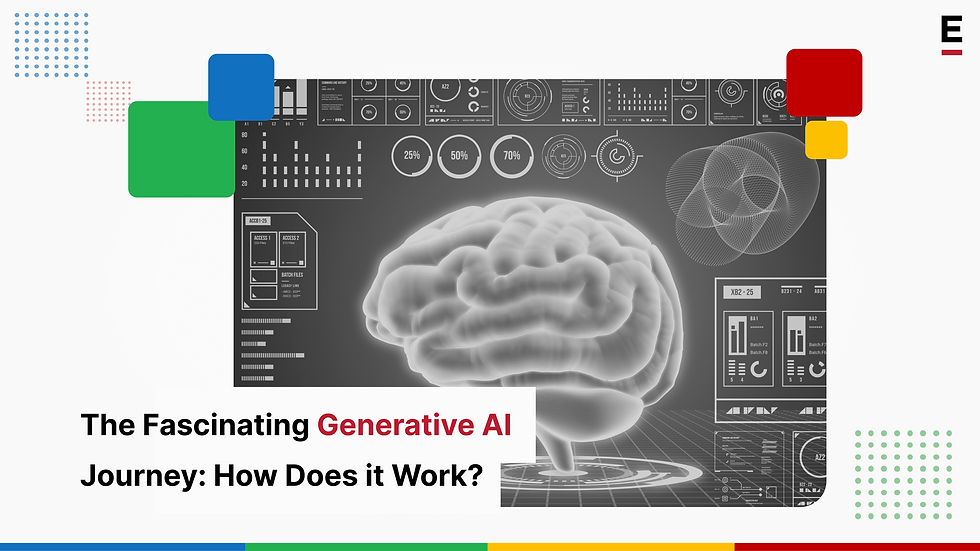
How Does Generative AI Work?
Generative AI represents a pivotal facet of Artificial Intelligence, a domain transcending conventional AI systems' mere analysis and decision-making capacities. Instead, Generative AI models are engineered to exceed these limitations by pioneering the creation of novel content or data, occasionally straying from the confines of their training data. These models have garnered widespread acclaim for their versatility, being proficient in tasks ranging from text generation and image synthesis to music composition and beyond. Delving deeper into the intricacies of Generative AI, let us explore its fundamental workings.
1. Data Collection and Preprocessing: At the inception of Generative AI model development, an indispensable initial step involves accumulating an extensive dataset. The chosen dataset should ideally mirror the type of data that the model is expected to generate. For instance, in the case of constructing a text generation model, curating a substantial corpus of text becomes imperative. Subsequently, this data undergoes preprocessing; meticulously cleaned, and formatted to align with the model's training requirements.
2. Choice of Model: Generative AI's toolbox boasts various models tailored to specific data types and generation objectives. Some of the foremost models include,
Recurrent Neural Networks (RNNs): These models excel in handling sequential data and are ideally suited for tasks like text generation and music composition.
Convolutional Neural Networks (CNNs): Primarily leveraged for image generation and style transfer, CNNs are indispensable for visual content creation.
Generative Adversarial Networks (GANs): GANs epitomize a groundbreaking paradigm comprising two neural networks — a generator and a discriminator — engaged in an adversarial duel. The generator strives to fabricate data indistinguishable from authentic data, while the discriminator endeavors to differentiate between genuine and generated data. GANs reign supreme in the realm of image and video generation.
Variational Autoencoders (VAEs): VAEs serve as a stalwart choice for data generation by masterfully learning a probabilistic model that underpins the data's inherent structure. Applications span from image generation to data compression.
3. Training the Model: The training phase is pivotal in the evolution of the generative AI model. Here, the model embarks on discerning intricate patterns and relationships within the training data. For instance, a text generation model acquires a nuanced understanding of grammatical structures, stylistic nuances, and content characteristics embedded within the text corpus it was nurtured on. Through iterative parameter adjustments, the model strives to minimize the discernible distinctions between its generated output and authentic data.
4. Sampling and Generation: Upon successful training, the generative AI model metamorphoses into a creative entity capable of producing fresh data samples. Sampling involves providing the model with a seed or initial input, triggering data generation based on this stimulus. The generated output manifests probabilistic attributes, rendering it variable across successive runs.
5. Fine-Tuning and Evaluation: Post-generation, fine-tuning the model to align the generated output with specific criteria or quality standards is often necessary. This iterative refinement process entails meticulous parameter adjustments while subjecting the output to rigorous evaluation against predefined metrics.
6. Deployment and Use Cases: Generative AI models have found their applications across many domains. Their significance extends to natural language generation, image synthesis, artistry generation, recommendation systems, and more. They serve as invaluable tools for automating content creation, fostering creativity, and augmenting decision-making processes.
7. Ethical Considerations: It is imperative to underscore that generative AI models occasionally yield biased, inappropriate, or inaccurate content. Ethical considerations loom large in their deployment, necessitating a vigilant approach to ensure responsible and safe utilization of this transformative technology. Researchers and developers remain committed to addressing these challenges and upholding the tenets of ethical AI deployment.
As we explore how generative AI works and its fundamental processes, it is essential to connect these insights with the fascinating history of this technology. The story of generative AI is a journey filled with remarkable moments and discoveries that have brought us to where we are today in 2023. So, let us transition from understanding how it operates to tracing its evolution, from its early beginnings to the latest advancements. What is the History of Generative AI (1932-2023)?
Generative AI has evolved remarkably over the decades, transforming from rudimentary experiments to a powerful force in Artificial Intelligence and creative content generation. This section explores the pivotal moments and innovations that have defined Generative AI's journey up to 2023.
1932-1960s: Early Foundations: The journey of generative AI began in 1932 when Georges Artsrouni invented a "mechanical brain" for language translation using punch cards. This early attempt laid the groundwork for future language generation technologies. In the late 1940s, the origins of Generative AI can be traced back to the early days of computing when researchers began exploring the idea of creating intelligent machines. Pioneers like Alan Turing laid the theoretical foundation for AI by proposing the concept of a "universal machine" that could simulate any human intelligence task. In the 1960s, MIT professor Joseph Weizenbaum created Eliza, the first chatbot, simulating conversations with a psychotherapist. These humble beginnings marked the inception of Generative AI.
1970s-1980s: Procedural Content Generation and Neural Networks: The late 1970s saw the emergence of procedural content generation in gaming with Don Worth's "Beneath Apple Manor." This technique programmatically created game worlds, foreshadowing Generative AI's role in entertainment. Moreover, in 1976, Mathematician and Architect Christopher Alexander authored “A Pattern Language”, which influenced architecture and inspired new software development. He published “Notes on the Synthesis of Form”, which states the principles for automating design that later influenced product parametric and generative design.
In 1989, Yann LeCun, Yoshua Bengio, and Patrick Haffner demonstrated Convolutional Neural Networks (CNNs) for image recognition, a breakthrough that would shape the future of image generation.
1990s-2000s: LSTMs, Bayesian Networks, and Word Vectors: Roger Schank's development of Conceptual Dependency Theory in the 1970s influenced natural language understanding and reasoning. The 1990s introduced Long Short-Term Memory (LSTM) architectures, developed by Sepp Hochreiter and Jurgen Schmidhuber, addressing the limitations of Recurrent Neural Networks (RNNs) for text generation. Bayesian network causal analysis by Judea Pearl offered ways to represent uncertainty, a crucial aspect in content generation. In 2006, Fei-Fei Li's ImageNet database fueled advancements in visual object recognition, setting the stage for image generation.
The 2010s: Deep Learning and Chatbots: The 2010s witnessed the rise of deep learning, particularly Convolutional Neural Networks (CNNs) and Recurrent Neural Networks (RNNs). In 2012, Alex Krizhevsky's AlexNet introduced deep learning scaling, significantly improving image recognition and generation. Meanwhile, Google's Tomas Mikolov introduced word2vec in 2013, revolutionizing natural language understanding and text generation.
Late 2010s: GANs, Transformers, and GPT: In 2014, Ian Goodfellow developed Generative Adversarial Networks (GANs), where two neural networks compete to generate and discriminate content, leading to significant advancements in image synthesis and content creation. In 2015, Stanford researchers published work on diffusion models in the paper "Deep Unsupervised Learning using Nonequilibrium Thermodynamics." The technique provided a way to reverse engineer the process of adding noise to a final image. It synthesizes pictures and videos, generates text, and models languages. Google introduced the transformative "Attention is all you need" paper in 2017, which inspired models like Transformers and BERT. OpenAI's GPT (Generative Pre-trained Transformer) models, starting with GPT-2 in 2019 and GPT-3 in 2020, became pioneers in natural language generation, chatbots, and content creation.
2020s: Image Synthesis and Multimodal AI: In 2021, OpenAI introduced DALL-E, capable of generating images from text prompts, highlighting the potential of generative AI in the visual arts. In 2022, DALL-E 2 was released, emphasizing efficiency and image generation through diffusion models.
2023: The Rise of Multimodal Generative AI: In early 2023, OpenAI unveiled GPT-4, a multimodal Large Language Model (LLM) capable of receiving text and image prompts. This marked a significant leap in Generative AI's capabilities, allowing for more versatile and creative content generation. The launch of GPT-4 spurred a global conversation about advanced AI systems' ethical and practical implications.
Generative AI has come a long way since its inception in the 1930s. From early language translation machines to the latest multimodal models like GPT-4, it has redefined content creation, natural language understanding, and image synthesis. As we look ahead, Generative AI continues to shape industries, artistic expression, and human-AI interaction, inviting excitement and contemplation about its limitless potential. In the next blog post, we will discuss the Generative AI models that allow magic to happen. Stay tuned!
Read other Extentia Blog posts here!
Comments